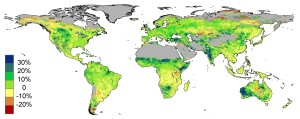
Estimated changes in vegetative cover due to CO2 fertilization between 1982 and 2010 (Donohue et al., 2013 GRL).
I testified before an administrative law judge (ALJ) in St. Paul, MN last week as part of a process that will determine what value the State of Minnesota decides to place on “carbon pollution”, also called the social cost of carbon (SCC).
This was the first expert testimony I have provided other than the several times I have testified in Congress. Congressional testimony is much more free-wheeling…more like a show for entertainment value and political posturing.
The Minnesota hearing was more like what you have seen on TV, with objections being made, sustained, and overruled. There were even accusations of “badgering the witness”.
It was interesting, to say the least.
There were economists who testified on both sides as to whether the economic models used were appropriate, whether they made valid assumptions, etc. I only saw two witnesses testify on that issue, one from each side.
It was clear that the lawyers from both sides were more comfortable cross-examining witnesses on economic issues than on the science behind the IPCC’s estimates of future warming, which (of course) are one of the primary inputs to any SCC model calculation. The greater the human-caused climate change, presumably the greater the damage caused by it (although one can also claim there are benefits, since cold weather kills more people on average than hot weather).
The judge had another judge present to help her out, one with an economics background and who could advise the ALJ on some of the more technical issues. The ALJ seemed most focused on procedural issues (as she should be, I suppose), making decisions regarding whether certain pieces of evidence would be admitted, etc. She seemed fair in the way she handled objections from both sides.
Scientists providing 5-minute opening statements along with me were Dick Lindzen and Will Happer. Lindzen mainly addressed climate sensitivity, Happer argued that CO2 emissions were actually a benefit, and I emphasized that the IPCC models used for the SCC calculations were demonstrably biased in their global warming projections.
As I recall, Happer received a minor question on cross-examination, while Lindzen was pressed on one of his claims regarding climate sensitivity, which he was forced to clarify. All five lawyers declined to ask me any questions on cross examination.
All of us provided written testimony well in advance of the hearing, which was responded to with rebuttal testimony from Andy Dessler and John Abraham. We also provided written rebuttal testimony in response toDessler’s and Abraham’s original written testimony. Another round of surrebuttal testimony then ensued. I believe that Dessler and Abraham provided opening statements this week, but I haven’t heard how that went.
Minnesota state law apparently requires there to be a social cost of carbon assigned to energy production in the state. I suppose that, theoretically, the assigned value could be zero. The question for the judge to address now is whether to replace the current value(s) [which are claimed by environmentalists to be too low] with the federal value, which is much higher, or whether it should be recalculated from scratch. Some good background on this can be found in a news story here from a year ago.
No matter which way the judge rules, I hear the ruling will likely be appealed. Then, no matter what the final ruling is, the Minnesota Public Utilities Commission can probably just do what they want to do, anyway. I believe that the Commission simply asked the judge to help them with the process. I will admit that legal issues sometimes confuse me, so people are free to correct me on any of this I got wrong.
I suspect we are going to see more state-level challenges to the “social cost of carbon”, which is basically addressing the unintended “negative externality” consequences of our use of carbon-based fuels. My opinion is that there has been no demonstrated damage caused by adding 1 carbon dioxide molecule to 10,000 molecules of air over the last 100 years. Even the IPCC admits the evidence for increased severe weather is shaky, at best. Whether sea level rise is greater than it was before CO2 emissions could contribute is also debatable.
We do, however, have evidence that increased CO2 boosts crop production and has led to global greening, a positive externality. So, I have to wonder whether the social cost of carbon is actually negative.
My suspicion is that we are in for years of debate and legal challenges on this issue. It seems like the social cost of carbon is an unusual case for the environmentalists to make, when the supposed damages caused by CO2 emissions are not really demonstrable, and future damages are largely theoretical.