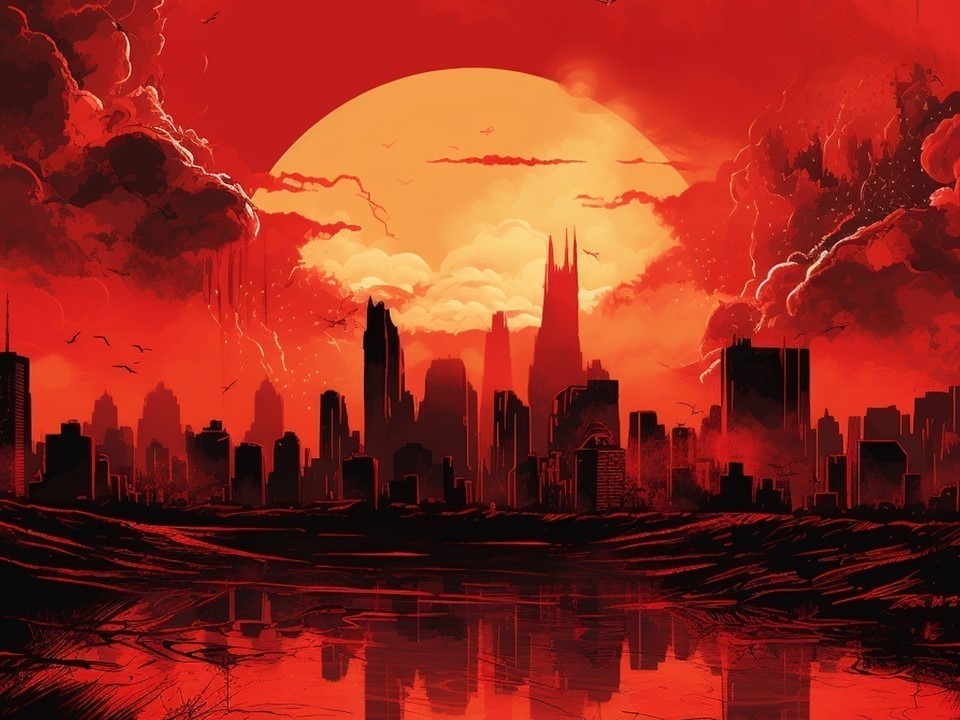
Since few people who visit here will actually download and analyze data, I present some imagery of the new Urban Heat Island (UHI) dataset we have developed, at their full (~9×9 km or better) spatial resolution.
A Review: The Method
(Skip this section if you just want to see the pretty pictures, below).
To review, the dataset is based upon over 13 million station-pairs of monthly average air temperature measurements at closely-spaced GHCN stations between 1880 and 2023. It quantifies the average *spatial* relationship between 2-station differences in temperature and population density (basically, quantifying the common observation that urban locations are warmer than suburban, which are in turn warmer than rural). The quantitative relationships are then applied to a global population density dataset extending back through time.
The quantitative relationships between temperature and population are almost the same whether I use GHCN raw or adjusted (homogenized) data, with the homogenized data producing a somewhat stronger UHI signal. They are also roughly the same whether I used data from 1880-1920, or 1960-1980; for this global dataset, all years (1880 through 2023) are used together to derive the quantitative relationships.
I use six classes of station-pair average population density to construct the (nonlinear) relationship between population density and the UHI effect on air temperature. To make the UHI dataset, I apply these equations (derived separately in 7 latitude bands and 4 seasons) to global gridded population density data since 1800.
As I previously announced, our paper submitted for publication on the method showed that UHI warming in the U.S. since 1895 is 57% of the GHCN warming trend averaged over all suburban and urban stations. But because most of the U.S. GHCN stations that go into the CONUS area average are rural, the UHI warming trend area averaged across all GHCN stations is only 20% of that computed from GHCN data. Thus, there is evidence that GHCN warming trends for the U.S. as a whole have been inflated somewhat (20% or so) by the urban heat island effect, but by a much larger fraction at urban station locations. The UHI contamination of the area average trends could be larger than this, since we do not account for some regions possibly having increased levels of UHI contamination as prosperity increases (more buildings, pavement, vehicles, air conditioning, and other waste heat sources) increases but population remains the same.
Some Dataset Examples
Here are some examples of the UHI dataset for several regions, showing the estimated total UHI effect on air temperature in the years 1850 and 2023 (I have files every 10 years from 1800 to 1950, then yearly thereafter). By “total UHI effect” I mean how much warmer the locations are compared to wilderness (zero population density) conditions. I emphasize the warm season months, which is when the UHI effect is strongest.
Remember, these quantitative relationships hold for the *average* of all GHCN stations in 7 separate latitude bands. It is unknown how accurate they are at individual locations depicted in the following imagery.
First let’s start with a global image for April, 2023 that Danny Braswell put together for me using mapping software, for April of 2023 (click on the image for higher resolution… and if you dare, here is a super-duper-hi-res version):
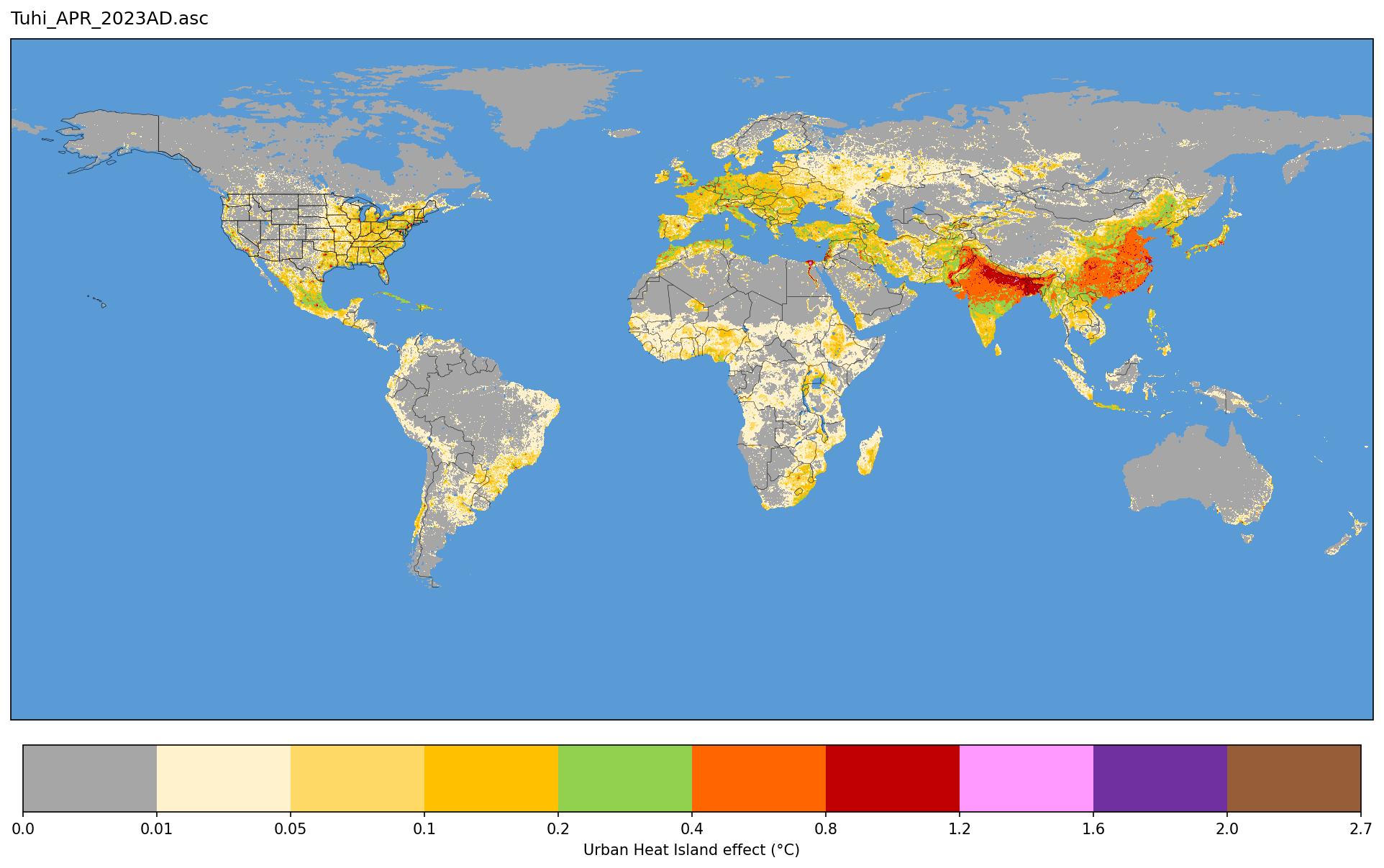
And here are some regional images using my crude Excel “mapping” (no map outlines):
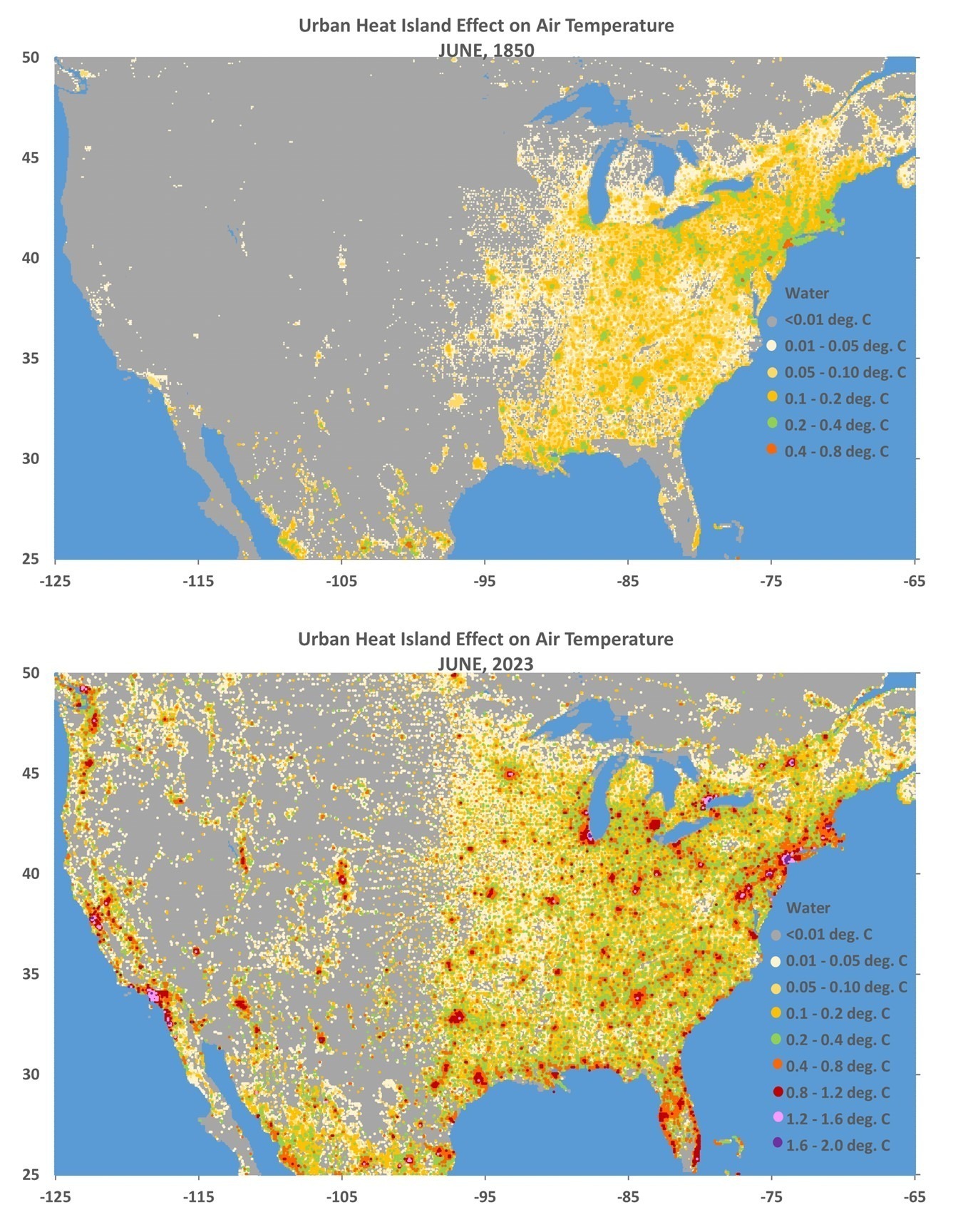
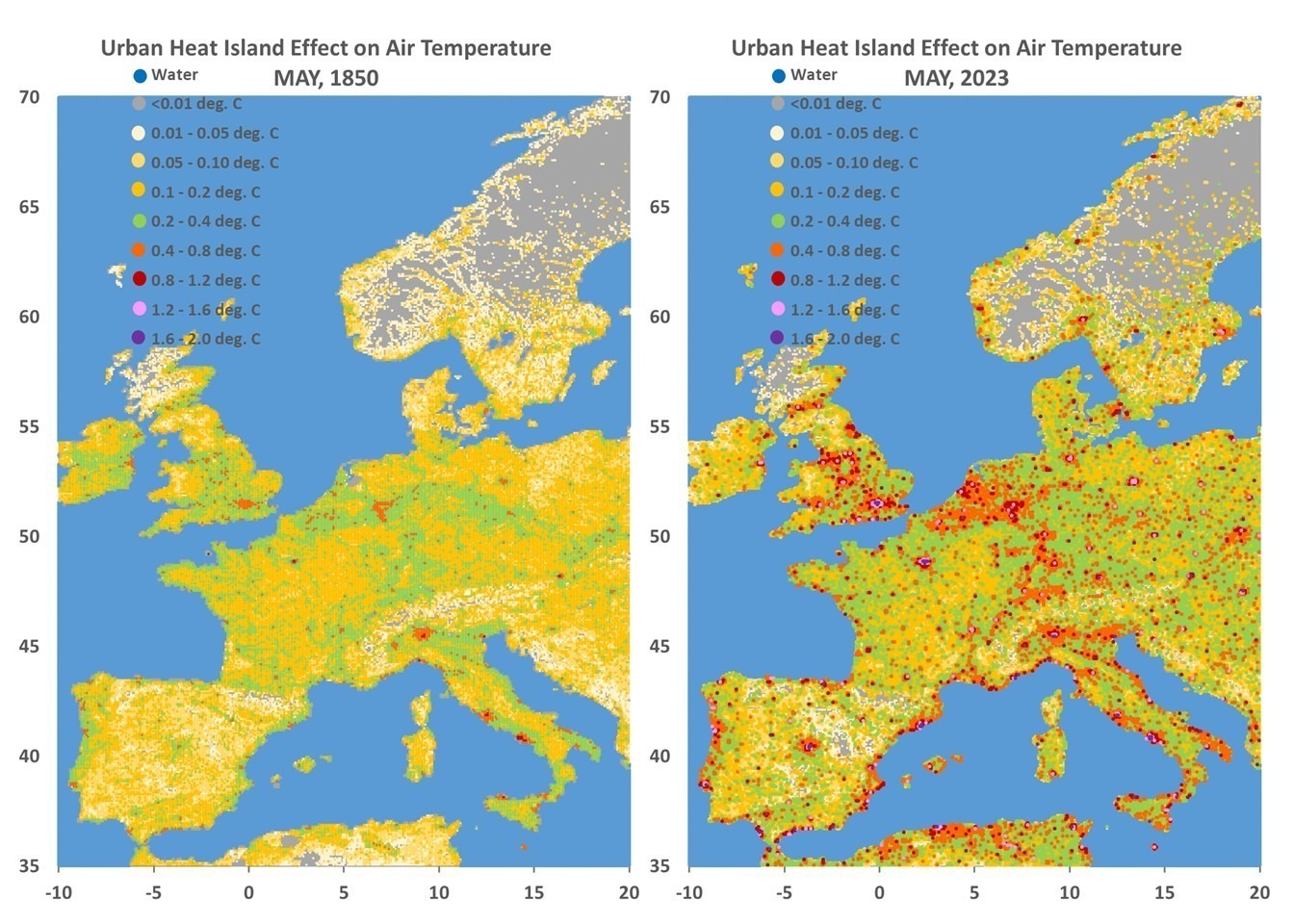
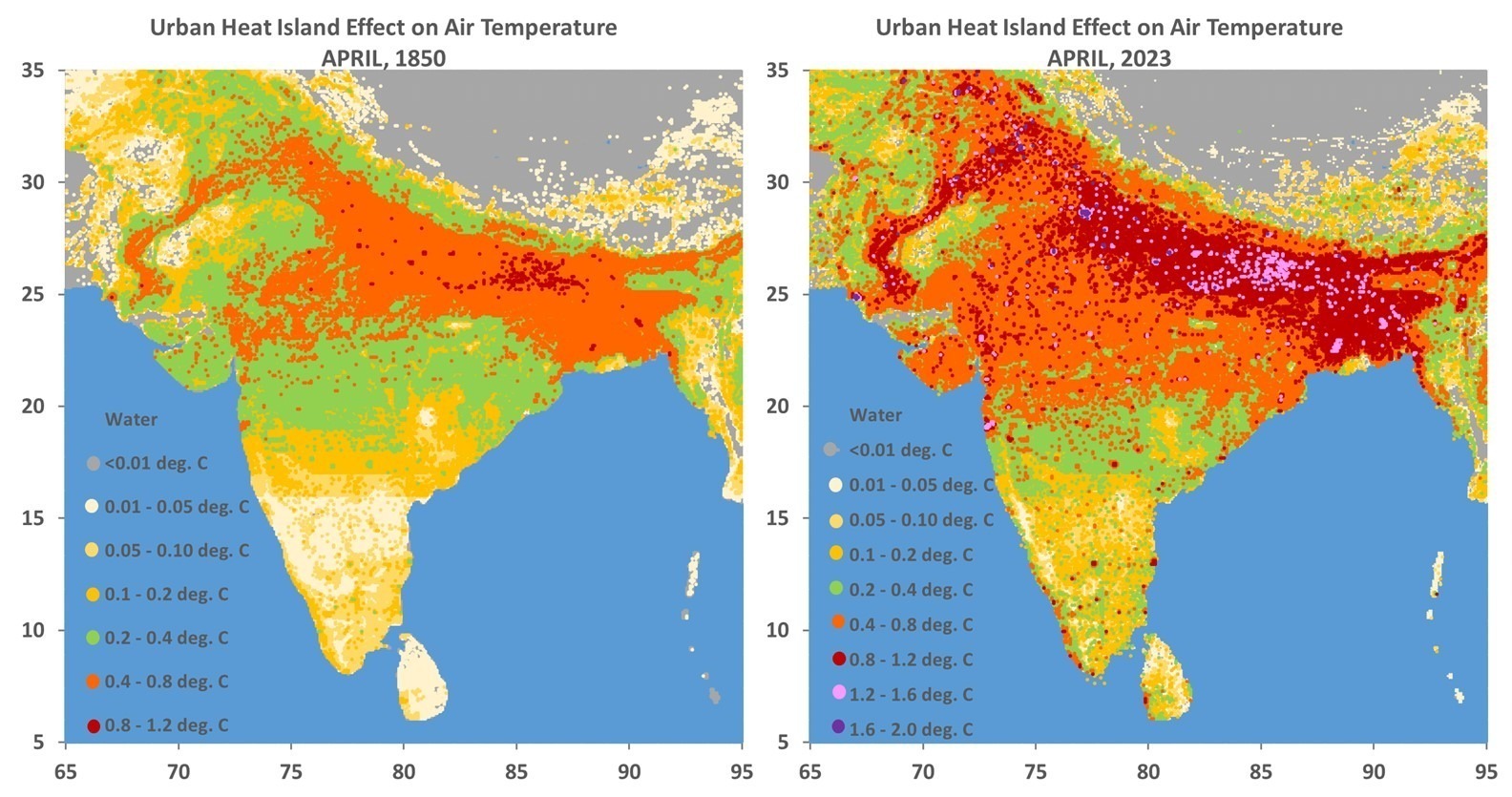
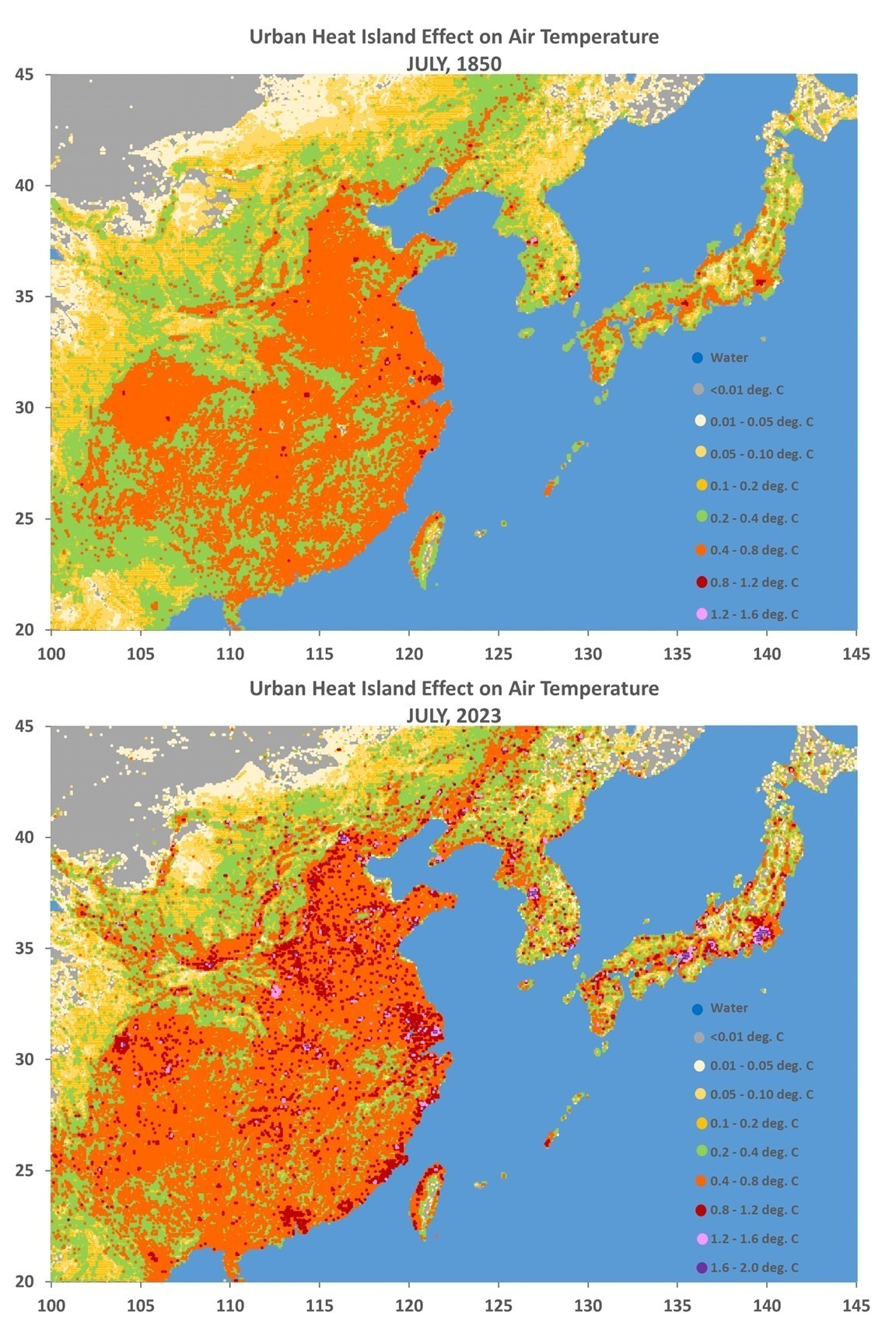
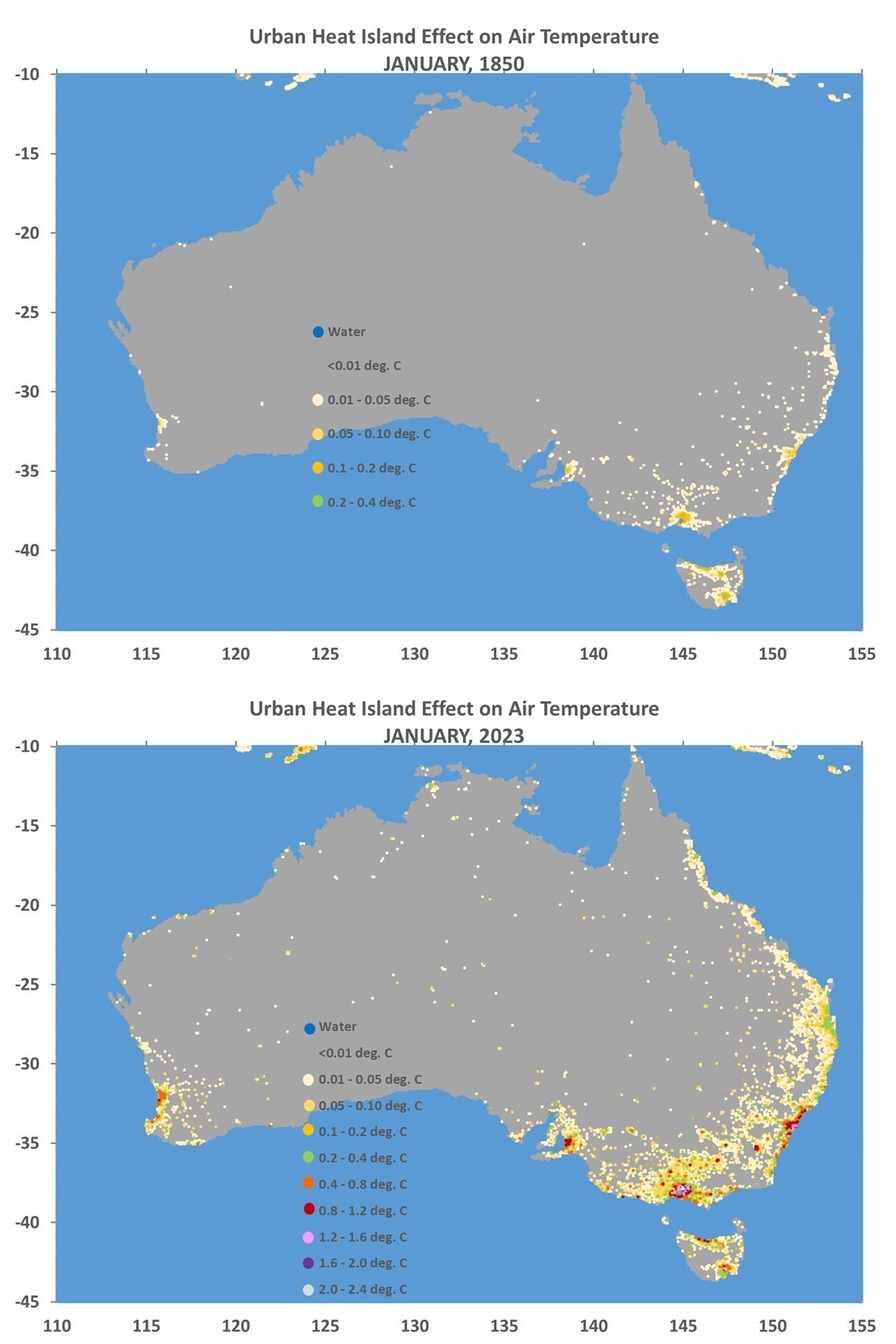
In my next post I will probably do some graphs of just how many people in the world live in various levels of elevated temperature just because the global population is increasingly urbanized. Over 50% of the population now lives in urban areas, and that fraction is supposed to approach 70% by 2045. This summer we have seen how the media reports on temperature records being broken for various cities and they usually conflate urban warmth with global warming even through such record-breaking warmth would increasingly occur even with no global warming.
Again, all of the ArcGIS format (ASCII grid) files are located here (public permissions now fixed).