by John R. Christy and Roy W. Spencer
University of Alabama in Huntsville
A new paper by Stephen Po-Chedley and Quang Fu (2012) (hereafter PCF) was sent to us at the end of April 2012 in page-proof form as an article to appear soon in the Journal of Atmospheric and Oceanic Technology. The topic of the paper is an analysis of a single satellite’s impact on the rarely-used, multi-satellite deep-layer global temperature of the mid-troposphere or TMT. Some of you have been waiting for our response, but this was delayed by the fact that one of us (J. Christy) was out of the country when the UW press release was issued and just returned on Tuesday the 8th.
There are numerous incorrect and misleading assumptions in this paper. Neither one of us was aware of the paper until it was sent to us by Po-Chedley two weeks ago, so the paper was written and reviewed in complete absence of the authors of the dataset itself. In some cases this might be a normal activity, but in a situation where complicated algorithms are involved, it is clear that PCF did not have a sufficient understanding of the construction methodology.
By way of summary, here are our main conclusions regarding the new PCF paper:
1) the authors’ methodology is qualitative and irreproducible
2) the author’s are uninformed on the complexity of the UAH satellite merging algorithm
3) the authors use the RSS (Remotes Sensing Systems) satellite dataset as “verification” for their proposed UAH NOAA-9 calibration target adjustment for TMT, but barely mention that their TLT (lower tropospheric) results are insignificant and that trends are essentially identical between UAH and RSS without any adjustment in the NOAA-9 calibration coefficient
4) the authors neglected the main TMT differences among the datasets – and instead try to explain the UAH v. RSS trend difference by only two years of NOAA-9 data, while missing all of the publications which document other issues such as RSS problems with applying the diurnal correction.
The paper specifically claims to show that a calibration target coefficient of one satellite, NOAA-9, should be a value different than that calculated directly from empirical data in UAH’s version of the dataset. With an adjustment to the time series guesstimated by PCF, this increases the UAH overall global trend by +0.042 °C/decade. Their new UAH trend, being +0.042 warmer, then becomes the same as the TMT trend from RSS. This, they conclude, indicates a verification of their exercise.
More importantly, with regard to the most publicized UAH dataset, the temperature of the lower troposphere (TLT), there was no similar analysis done by PCF – an indication that their re-calculations would not support their desired outcome for this dataset, as we shall demonstrate below.
All of this will soon be moot, anyway. Since last year we have been working on v6.0 of the UAH datasets which should be ready with the tropospheric temperature datasets before summer is out. These will include (1) a new, more defensible objective empirical calculation to correct for the drift of the satellites through the diurnal cycle, and (2) a new hot calibration target effective emissivity adjustment which results in better agreement between simultaneously operating satellites at the calibration step, making the post-calibration hot-target adjustment PCF criticizes unnecessary. So, since our new v6.0 dataset is close to completion and submission for publication, we have chosen this venue to document PCF’s misinformation in a rather informal, but reproducible, way rather than bother to submit a journal rebuttal addressing the older dataset. However, to show that version 5.4 of our datasets was credible, we discuss these issues below.
The Lower Tropospheric Temperatures (TLT)
We shall return to TMT below, but most of the research and popular use of the UAH datasets have focused on the lower tropospheric temperature, or TLT (surface to about 300 hPa, i.e. without stratospheric impact). Thus, we shall begin our discussion with TLT because it is rightly seen as a more useful variable because it documents the bulk heat content of the troposphere with very little influence from the stratosphere. And [this is important in the TMT discussion] the same hot-target coefficients for NOAA-9 were used in TLT as in TMT.
PCF focused on the deep layer TMT, i.e. temperature of the surface to about 75 hPa, which includes quite a bit of signal above 300 hPa. As such, TMT includes a good portion of the lower stratosphere – a key weakness when utilizing radiosondes which went through significant changes and adjustments during this time. [This was a period when many stations converted to the Vaisala 80 radiosonde which introduced temperature shifts throughout the atmosphere (Christy and Norris 2004).]
As indicated in their paper, it seems PCF’s goal was to explain the differences in trend between RSS and UAH, but the history of this effort has always been to find error with UAH’s products rather than in other products (as we shall see below). With us shut out of the peer-review cycle it is easy to assume an underlying bias of the authors.
Lord Kelvin told us that “All science is numbers”, so here are some numbers. First, let’s look at the “global” trends of UAH and RSS for TLT (70S to 82.5N) for Jan 1979 to Apr 2012:
+0.137 °C/decade UAH LT (70S-82.5N)
+0.134 °C/decade RSS LT (70S-82.5N)
These trends are, for all practical purposes, identical. This, however, hides the fact that there are indeed differences between the two time series that, for one reason or another, are balanced out when calculating the linear trend over the entire 30+ year period. As several papers have documented (see Christy et al. 2011, or C11, for the list – by the way, C11 was not cited by PCF) the evidence indicates RSS contains a spurious warming in the 1990’s then a spurious cooling from around 2002 onward (note that the RSS temperature anomaly for last month, April, 2012, was 0.08°C cooler than our UAH anomaly).
This behavior arises, we believe, from an over-correction of the drift of the satellites by RSS (in the 1990’s the satellites drifted to cooler times of day, so the correction must add warming, and in the 2000’s the satellites drifted to warmer times of day so a correction is needed to cool things down.) These corrections are needed (except for the Aqua satellite operating since 2002, which has no diurnal drift and which we use as an anchor in the UAH dataset) but if not of the right magnitude they will easily affect the trend.
In a single paragraph, PCF admit that the UAH TLT time series has no significant hot-target relationship with radiosonde comparisons (which for TLT are more robust) over the NOAA-9 period. However, they then utilize circular reasoning to claim that since RSS and UAH have a bit of disagreement in that 2-year period, and RSS must be correct, that then means UAH has a problem. So, this type of logic, as stated by PCF, points to their bias – assume that RSS is correct which then implies UAH is the problem. This requires one to ignore the many publications that show the opposite.
Note too that in their press release, PCF claim that observations and models now are closer together for this key parameter (temperature of the bulk troposphere) if one artificially increases the trend in UAH data. This is a questionable claim as evidence shows TLT for CMIP3 and CMIP5 models averages about +0.26 °C/decade (beginning in 1979) whereas UAH *and* RSS datasets are slightly below +0.14 °C/decade, about a factor of 2 difference between models and observations. We shall let the reader decide if the PCF press-release claim is accurate.
The key point for the discussion here (and below) is that TLT uses the same hot-target coefficients as TMT, yet we see no problem related to it for the many evaluation studies we have published. Indeed this was the specific result found in Christy and Norris 2004 – again, work not cited by PCF.
The Mid-Tropospheric Temperature (TMT)
About 12 years ago we discovered that even though two different satellites were looking at the same globe at the same time, there were differences in their measurements beyond a simple bias (time-invariant offset). We learned that these were related to the variations in the temperature of the instrument itself. If the instrument warmed or cooled (differing solar angles as it orbited or drifted), so did the calculated temperature. We used the thermistors embedded in the hot-target plate to track the instrument temperature, hence the metric is often called the “hot target temperature coefficient.”
To compensate for this error, we devised a method to calculate a coefficient that when multiplied by the hot target temperature would remove this variation for each satellite. Note that the coefficients were calculated from the satellite data, they were not estimated in an ad hoc fashion.
The calculation of this coefficient depends on a number of things, (a) the magnitude of the already-removed satellite drift correction (i.e. diurnal correction), (b) the way the inter-satellite differences are smoothed, and (c) the sequence in which the satellites are merged.
Since UAH and RSS perform these processes differently, the coefficients so calculated will be different. Again recall that the UAH (and RSS) coefficients are calculated from a system of equations, they are not invented. The coefficients are calculated to produce the largest decrease in inter-satellite error characteristics in each dataset.
To make a long story short, PCF focused on the 26-month period of NOAA-9 operation, basically 1985-86. They then used radiosondes over this period to estimate the hot-target coefficient as +0.048 rather than UAH’s calculated value of +0.0986. [Note, the language in PCF is confusing, as we cannot tell if they conclude our coefficient is too high by 0.051 or should actually be 0.051. We shall assume they believe our coefficient is too high by 0.051 to give them the benefit of the doubt.]
Recall, radiosondes were having significant shifts with the levels monitored by TMT primarily with the switch to Vaisala 80 sondes, and so over small, 26-month periods, just about any result might be expected. [We reproduced PCF’s Fig. 2 using only US VIZ sondes (which had no instrument changes in the 26-month period and span the globe from the western tropical Pacific to Alaska to the Caribbean Sea) and found an explained variance of less than 4% – an insignificant value.]
Another problematic aspect of PCF’s methodology is that when looking at the merged time series, one does not see just NOAA-9’s influence, but the impact of all of the other satellites which provided data during 1985-86, i.e. NOAA-6, -7 and -8 as well. So, it is improper to assume one may pick out NOAA-9’s impact individually from the merged satellite series.
That PCF had little understanding of the UAH algorithm is demonstrated by the following simple test. We substituted the PCF value of +0.048 directly into our code. The increase in trend over our v5.4 TMT dataset was only +0.022 °C/decade for 1979-2009 (not 0.042), and +0.019 °C/decade for 1979-2012.
To put it another way, PCF overestimated the impact of the NOAA-9 coefficient by a factor of about 2 when they artificially reconstructed our dataset using 0.048 as the NOAA-9 coefficient. In fact, if we use an implausible target coefficient of zero, we still can’t return a trend difference greater than +0.037 °C/decade. Thus PCF have incorrectly assumed something about the construction methodology of our time series that gave them a result which is demonstrated here to be faulty.
In addition, by changing the coefficient to +0.048 in an ad hoc fashion, they create greater errors in NOAA-9’s comparisons to other satellites. Had they contacted us at any point about this, we would have helped them to understand the techniques. [There were 4 emails from Po-Chedley in Aug and Sep 2011, but this dealt with very basic facts about the dataset, not the construction methodology. Incidently, these emails were exchanged well after C11 was published.]
PCF brought in a third dataset, STAR, but this one uses the same diurnal corrections and sequential merging methodology as RSS, so it is not a truly independent test. As shown in C11, STAR is clearly the outlier for overall trend values due to a different method of debiasing the various satellite data and a differing treatment of the fundamental brightness temperature calibration.
We have additional information regarding UAH’s relatively low error statistics. Using radiosondes to evaluate microwave temperatures requires great care. In our tests, we concentrated on sondes which had documented characteristics and a high degree of consistency such as the US VIZ and Australian sondes. These comparisons have been published a number of times, but most recently updated in C11.
Here are the comparisons for the US VIZ radiosonde network (stretching from the western tropical Pacific to Alaska down across the conterminous US and to the Caribbean.) As you can see, UAH MT provides the lowest error magnitudes and highest reproducibility of the three data sets. Similar results were found for the Australian comparisons.
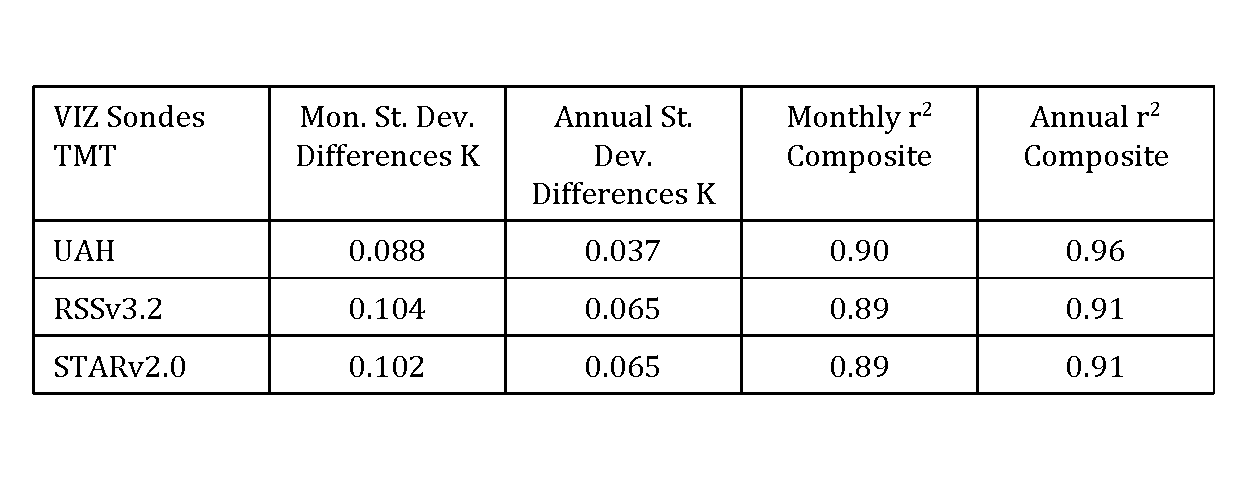
For data through April 2012 we have the following global TMT trends: UAH +0.045, RSS +0.079 and STAR +0.124 °C/decade. So, RSS, in the middle, is closer to UAH than STAR, yet PCF chose to examine UAH as the “problem” dataset. Had PCF wanted to pick some low-hanging fruit regarding the differences between UAH, RSS and STAR, they would have (a) looked at the diurnal differences between UAH and RSS (see publications) or (b) looked at a simple time series of differences between the three datasets (below). One thing that pops out is a spurious upward shift in STAR TMT relative to UAH and RSS of about +0.06 °C on precisely 1 Jan 2001 – an obvious beginning-of-year glitch. Why not look there?
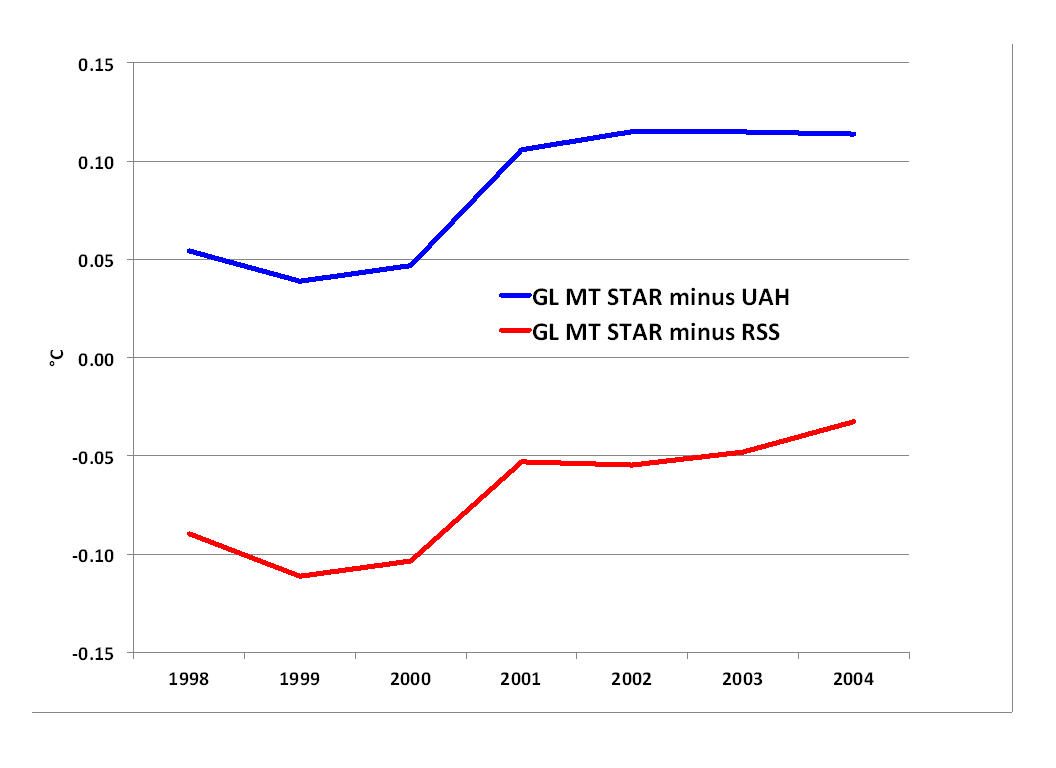
The Bottom Line
In conclusion, we believe that the result in PCF was a rather uninformed attempt to find fault with the UAH global temperature dataset, using an ad hoc adjustment to a single, short-lived satellite while overlooking the greater problems which have been documented (published or as demonstrated in the figure above) regarding the other datasets.
And think about this. If PCF is correct that we should be using a revised NOAA-9 coefficient, and since we use the same coefficient in both TMT and TLT, then the near perfect agreement currently between RSS and UAH for TLT will disappear; our TLT trend will become warmer, and then RSS will have the lowest warming trend of all the satellite datasets. The authors of the new study cannot have it both ways, claiming their new adjustment brings RSS and UAH closer together for TMT (a seldom used temperature index), but then driving the UAH and RSS trends for TLT farther apart, leaving RSS with essentially the same warming trend that UAH had before.
Since it is now within 3 months of the publication cutoff for research to be included in the IPCC AR5, one is tempted to conclude that PCF will be well-received by the Lead Authors (some of whom are closely associated with the RSS dataset) without critical evaluation such as briefly performed here. However, we cannot predict what the AR5 outcome will be or, for that matter, what waning influence the IPCC might still exert.
That PCF brushed aside the fact that the UAH and RSS trends for the LOWER troposphere are essentially identical (for which the UAH NOAA-9 coefficient is the same) seems to us to be a diversionary tactic we have seen before: create a strawman problem which will allow the next IPCC report to make a dismissive statement about the validity of an uncooperative dataset with a minimum of evidence. We hope that rationality instead prevails.
References
Christy, J.R. and W. B. Norris, 2004: What may we conclude about global tropospheric temperature trends? Geophys. Res. Lett. 31, No. 6.
Christy, J.R., R.W. Spencer and W.B Norris (deceased), 2011: The role of remote sensing in monitoring global bulk tropospheric temperatures. Int. J. Remote Sens. 32, 671-685, DOI:10.1080/01431161.2010.517803.
Po-Chedley, S. and Q. Fu, 2012: A bias in the midtropospheric channel warm target factor on the NOAA-9 Microwave Sounding Unit. J. Atmos. Oceanic Tech. DOI: 10.1175/JTECH-D-11-00147.1.